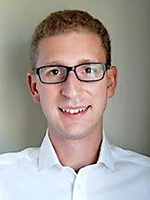
Raphael Stern, Assistant Professor, Civil, Environmental, and Geo- Engineering
Area of Expertise: Traffic Engineering & Management
Vehicle automation has the potential to fundamentally change how we think about transportation. Recent research findings have shown that automated vehicles (AVs) may reduce travel time, improve traffic safety, and, if equipped with connectivity for vehicle-to-vehicle or vehicle-to-infrastructure communication, even eliminate the need for traditional traffic control infrastructure such as traffic lights. This is primarily true for fully automated vehicles (often referred to as SAE Level 5), though recent research findings have shown that some of these benefits may soon be available with lower-level automation available in vehicles with advanced driver assistance systems (ADAS, often referred to as SAE Level 1-3). For example, ADAS features such as adaptive cruise control (ACC) automate part of the driving process by taking over speed and acceleration control (technically referred to as longitudinal control) of the vehicle.
...such attacks would actually adversely impact not only the attacked vehicle, but all other vehicles on the road as well, since they would all be stuck in the traffic jams these attacked vehicles trigger.
However, these automated (or partially automated) vehicles perform substantially more computation, and thus, provide more opportunities for malicious actors to compromise them. In technical terms, this is often referred to as the expansion of the attack surface. Even though vehicle manufacturers will clearly work to ensure that their vehicles are difficult to compromise, it will be difficult to fully ensure that no malicious code is embedded in the vehicle operating system, since the individual components of a vehicle are generally made by a number of distinct suppliers, who each may use a set of suppliers to assemble their components. Thus, the automotive industry may unintentionally be susceptible to supply-chain compromises, and malicious code could inadvertently end up on production vehicles.
Some types of compromises (colloquially referred to as hacks or attacks) may be catastrophic. For example, an attacker could potentially force vehicles to crash into other cars or into infrastructure components, risking passenger and pedestrian safety. However, such attacks would likely be straightforward to detect, since this type of behavior is far from typical. However, other more subtle attacks may be more difficult to detect but still damaging to overall transportation system efficiency. We generally refer to these attacks as stealthy cyberattacks, since they could be quite difficult to detect. For example, if an attacker compromises a subset of ADAS vehicles to brake and accelerate sporadically, this may be very difficult to detect, but could potentially trigger traffic waves and increase congestion and overall fuel consumption. Particularly concerning is that such attacks would actually adversely impact not only the attacked vehicle, but all other vehicles on the road as well, since they would all be stuck in the traffic jams these attacked vehicles trigger.
Over the past year, sponsorship through a seed grant from the University of Minnesota’s Center for Transportation Studies has enabled my research team to study these stealthy cyberattacks on AVs, quantify their impacts on the overall traffic, and develop methods to identify these attacks based on traffic data. Our findings show that these attacks will likely slow traffic speed and increase congestion for all vehicles on the road, without it being obvious which vehicle has caused congestion.
To identify if such an attack is occurring, we’ve studied the use of anomaly detection techniques to identify anomalous traffic flow. Specifically, we’ve used neural networks, a powerful machine learning tool, to build a model of what typical traffic should look like. Then, the model is used to identify how dissimilar the current observed traffic conditions are from what the neural network model predicts given the current underlying traffic flow conditions. If there is a big discrepancy, it is likely that some sort of anomalous conditions are present. However, this method does not pinpoint which vehicle is compromised. For that, we are currently working on vehicle-level anomaly detection, which will work in a similar way, but instead consider a model for individual vehicles and how they should react to their surroundings.
The research findings that have resulted from this seed grant have shown that the potential risk of stealthy cyberattacks is substantial. This work has highlighted the need for additional research in this area to identify exactly which vehicle has been compromised. Furthermore, simply knowing that a cyberattack has occurred is not sufficient. Future research must also identify a playbook for how to respond to a known cyberattack to minimize the overall societal risk and keep our transportation networks operating safely and efficiently.
July 2023